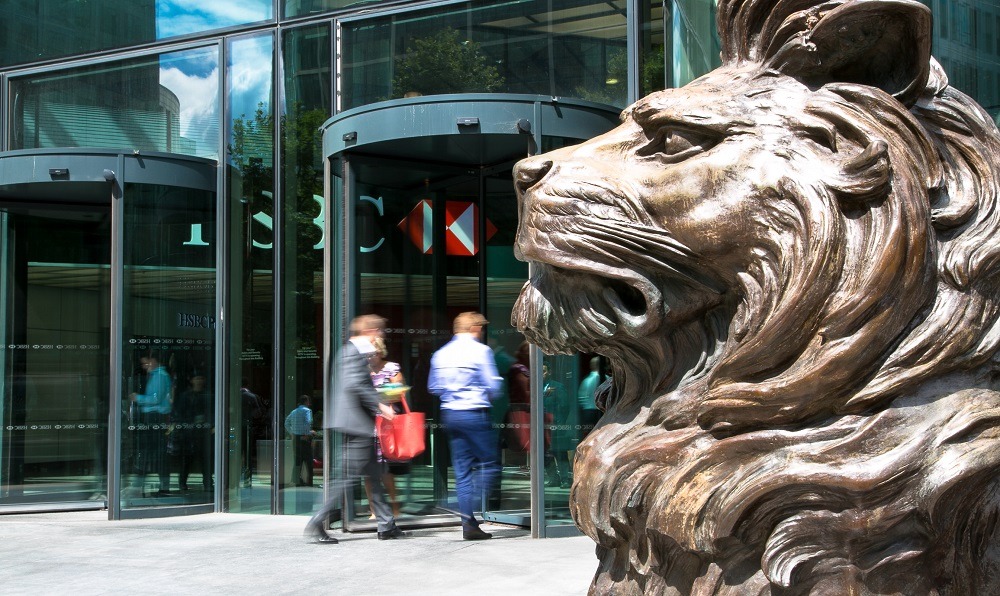
HSBC is using AI to help spot money laundering, fraud and terrorist funding. Future Banking writer Tim Gunn speaks to Marc Fungard, global head of research and analytics for the bank’s financial crime unit, to find out how the technology can aid in the fight against malicious activity.
If you want to understand his bank’s award-winning approach to combatting money laundering and criminal financing, HSBC’s Marc Fungard recommends you start watching old detective shows.
To save you 20 minutes, let’s role play. You’ve been transferred to run IT for an experimental investigative team, and you’re entering the office for the first time. There’s considerably more cigarette smoke than computer processing power. As you open the door, you’re met by a suitably grizzled chief who’s ushering someone – something? – out.
“Excuse the hype,” he says. “It’s just leaving.” There’s a beat of silence as the door shuts and the chief takes a slug of whisky. “Though this won’t be the last we’ll see of it,” he breathes. “Actually, why wait?” He snatches a ball of string and a pile of photos from a desk, and starts weaving thumbtack to thumbtack on the pinboard.
“It connects to all of this: AI, machine learning, big data, network analytics – and that last one might be even more important. I’ll be damned if I don’t recognise it from somewhere.”
Fungard does. “We’re working on a more intelligence-led approach to financial crime risk management,” he explains. “But a lot of the concepts that underpin this really aren’t new. Take network analytics, which is the idea that we can learn a lot about people by looking at who they interact with.
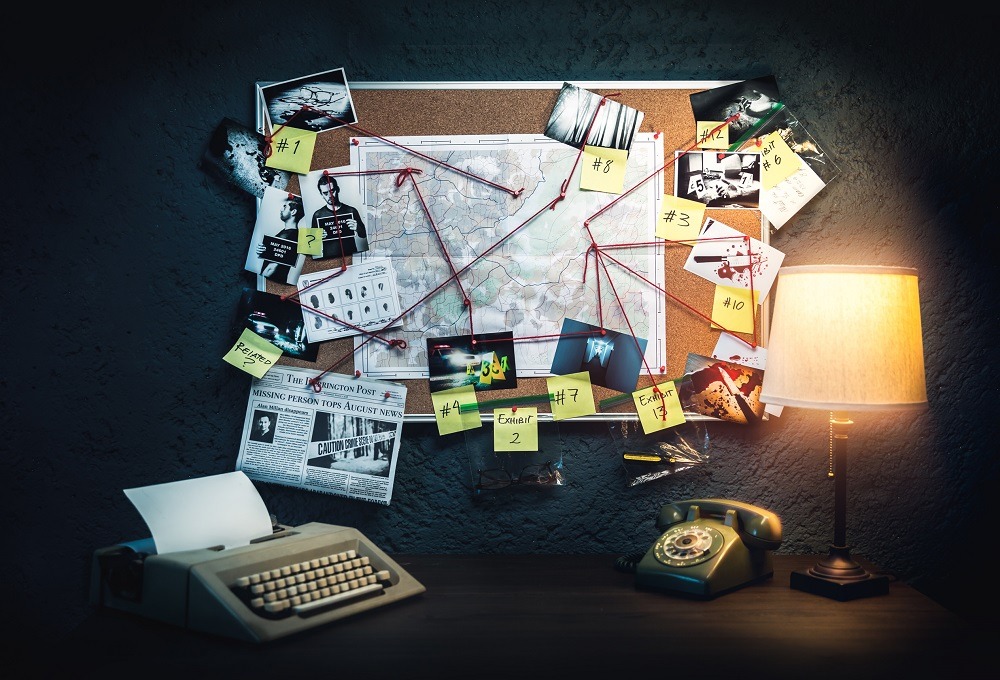
Now, if you think about all the crime shows that you’ve seen, where they’ve got the kingpin’s picture up on top and then they’re drawing all these connections with string – that’s network analytics. It’s being done in a manual way and quite slowly, but it’s network analytics.”
He would know. Fungard’s background is in investigating and analysing terrorism and financial crime for the US government. “Thinking about things like network analytics, but on a very different problem-set,” as he puts it.
HSBC has made a number of senior appointments in its efforts to fight financial crime
Given the value such a discipline places on context, it’s important to note that he’s one of a number of recent HSBC hires from US financial regulators. In 2016, the bank brought in Jennifer Shasky Calvery, Fungard’s former boss at the Treasury Department’s Financial Crimes Enforcement Network, as its global head of financial crime threat mitigation.
Remarkably, a few months after Fungard moved to London to help establish a global Financial Intelligence Unit for HSBC in 2012, Calvery was instrumental in fining the bank a then-record $1.92bn for failing to prevent Mexican and Colombian drug cartels laundering a total of $881m, among other compliance and due-diligence issues.
That and other fines imposed in the wake of the financial crisis, including an astonishing $8.9bn penalty given to BNP Paribas in 2014, certainly focused banks’ attention on regulatory compliance, but there have been complications.
As Calvery herself has acknowledged, there’s a perception that the regulators and the regulated aren’t the most comfortable bedfellows. The $26bn in penalties levied between 2008 and 2018 didn’t undercut that suspicion. Pushed onto the back foot just as legacy systems became problematic, banks implemented a defensive, rules-based approach to warding off risk.
As Fungard sees it, the problem with such rules is, “You either hit them or you don’t. And if you do, then all the machine says is, ‘You hit this rule.’”
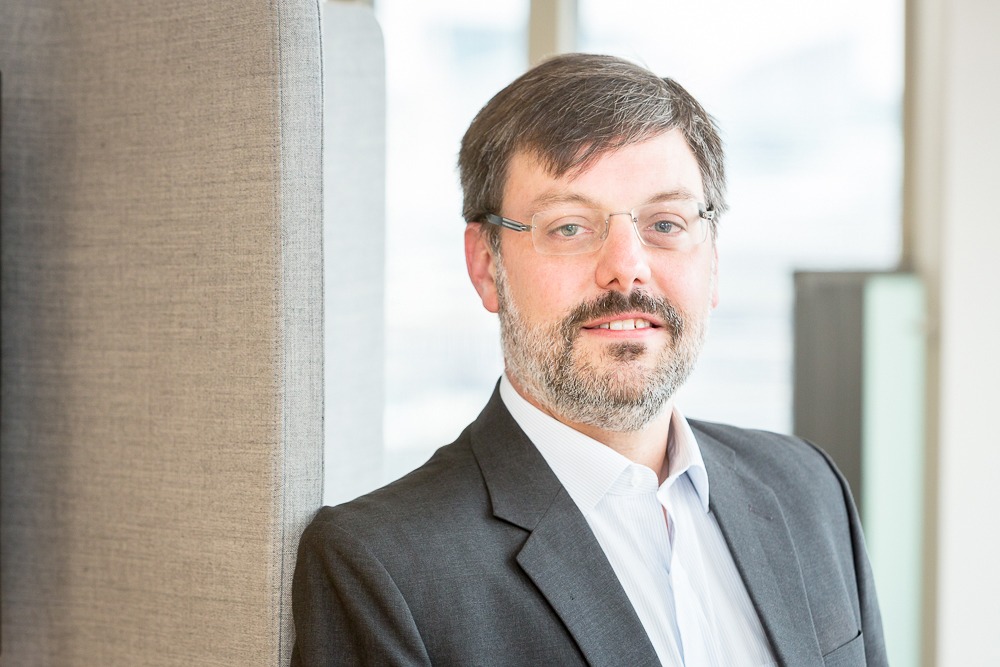
They can operate geographically, or by account type, but he gives the example of a mechanism that flags large round-number currency transactions as suspicious. “When individuals come around to bonus time,” he explains, “they very often hit on that rule. Lo and behold, it’s up to an investigator to gather all the information and conclude that they’ve got their bonus.”
The workload that comes with doing so is part of the reason that HSBC has quintupled the number of employees assigned to spot suspicious activity from 1,000 to 5,000 since 2012.
Major institutions’ use of such blunt tools also means that 95% of the financial industry’s system-generated anti-money-laundering alerts are closed as false positives in the first phase of review, and that smaller, less well-regulated banks have had to pick up the slack in risk-flagged countries.
HSBC has automated existing techniques for detecting signs of financial crime
When he was hired, Fungard focused on managing the investigative workload by applying network analytics. “We began to manually pull the data out of all the places that we could and combine it into a network to use in our investigations,” he explains. “Then over the course of several years we’ve automated how we do that and brought in more sophisticated techniques.”
Over time, rather than asking for investigators to follow-up on rules-based alerts by trawling records and databases for relevant information, HSBC has been able to move past static flagging procedures and apply AI and machine learning to ‘industrialise’ considerations of context into its systems.
Practically, their work may be focused on refining and developing risk management practices, but taken in context, hires like Fungard and Calvery, which have taken place across the world’s major financial institutions, have served to soften the borders between regulators and banks.
Indeed, despite her history with HSBC, Calvery explicitly rejects the premise of any question about switching teams. “The fact is,” she writes, “I’m on the same side as I always was.” She’s not there just to shield HSBC from risk, but to keep the banking system safe from financial crime.
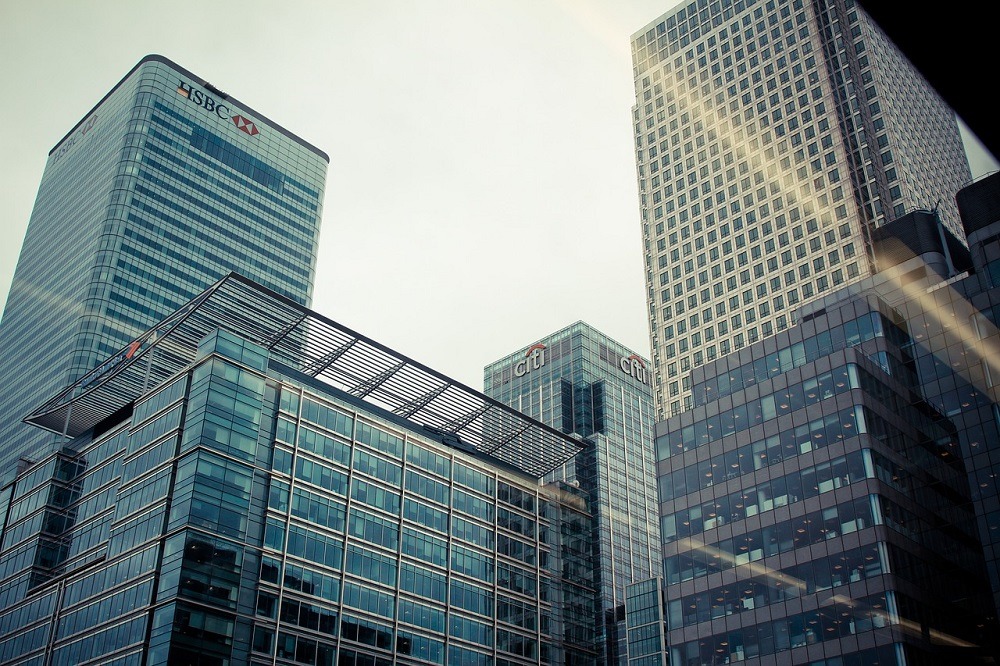
That way of thinking is reflected in her openness to discussing the problems the industry continues to face. “Despite all of the money we’re spending – and we’re spending a lot to keep criminal money out of our institutions – it’s still getting in every single day, right by all those controls,” Calvery told Bloomberg in April. “So we’re not doing as well as we’d like.”
HSBC has received recognition for its progress in tackling financial crime
Even so, HSBC is leading the pack. The bank’s Global Social Network Analytics (GSNA) platform won the 2019 Celent Model Bank Award for Risk Management. Developed in partnership with Quantexa, the initiative was adjudged “a significant step forward in the fight against financial crime for HSBC and the industry”.
As far as is possible given different data regulations around the world, the platform provides access to information from the more than 50 jurisdictions where the bank has a presence, giving HSBC’s investigators the ability to contextualise every customer by looking through their entire network and exploring the full extent of transaction flows and ownership structures across borders.
“HSBC holds many petabytes of data,” Fungard reveals. “Petabytes are big, right? And big becomes beautiful here, because that can tell us a lot. Take me; you could look at my accounts and discern pretty quickly that though I am an American, and though I have a US account, I am clearly active in London.
You could see that I live here and that this is where I would normally be. So if suddenly my US account got really active, that would be something we’d be interested in from a fraud perspective. “By bringing that context to the front, we’re much better able to make a decision as to whether that really is you touching your card to that thing, at that time, in that place.”
Payments are a key battleground in HSBC’s fight against financial crime
Another illustrative example of HSBC’s improved fraud-monitoring capabilities centres on the high-risk moment that an account makes a payment to a new recipient. The bank’s global scope and vast data reserves mean that, however new a recipient might be for an individual account, there’s a good chance that HSBC has dealt with them before.
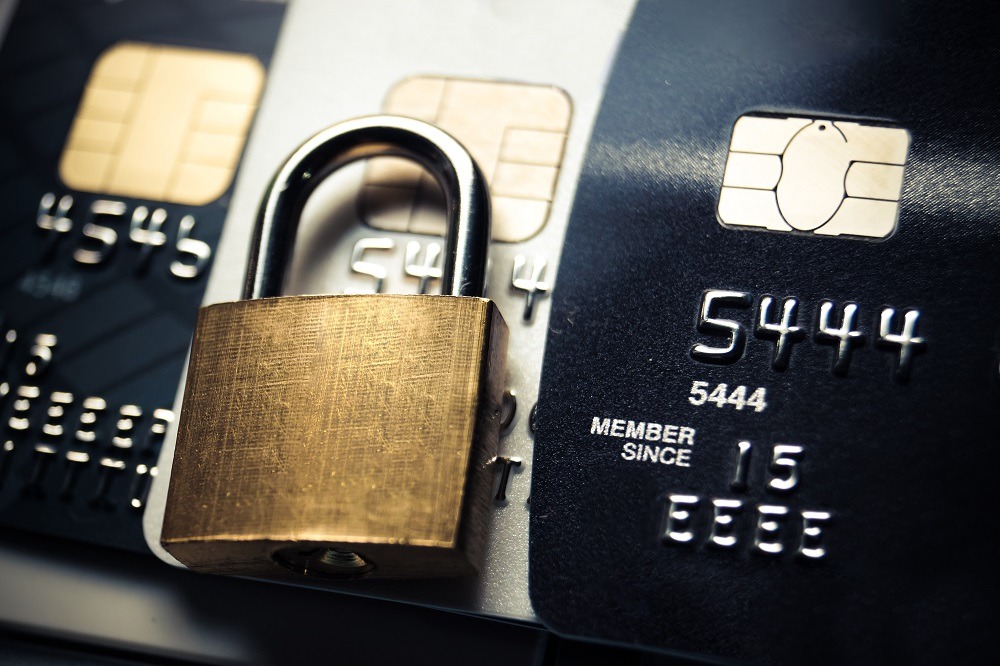
As such, Fungard points out, the bank is able to ask, “What do we know about this recipient? Have we seen them in other fraud cases? Is this payment going to purchase something that I know the recipient is a reliable purveyor of, and therefore I can be less concerned? Still, it’s important to remember that we have to comply with the relevant rules and legislation on data protection in every territory where we operate.”
That challenge granted, bringing context into consideration allows the bank to let legitimate payments through with minimal fuss, and focus investigative time and energy on transactions that are likely to be fraud, rather than bluntly stopping all payments that cross certain unresponsive boundaries.
On top of that, HSBC uses AI and machine learning to track and respond to far more subtle and revealing criminal typologies than unchanging rules ever could, revealing the patterned webs of transactions and behaviours that accompany crimes as complex and reprehensible as human trafficking or terrorist financing.
Human expertise remains an important aspect of financial crime investigation for HSBC
As the Celent Model Bank case study notes, by bringing all of this to an investigator’s attention, the GSNA platform has helped to reduce the length of a typical HSBC financial crime investigation from days to hours.
And as impressive as the technology is, the continuing importance of human expertise can’t be overstated. First, humans need to factor in the geopolitical situation to risk management, and keep track of how criminal behaviours change and adapt to prevent the automated system from simply cementing the biases of the past.
Then, after marshalling the data and treating it with automated analytics, the focus of any effective system is supporting the right decisions by delivering the relevant contextualised information to the right people.
“Do you want to begin immediate investigative action, or do you want a client relationship manager to start a conversation?” asks Fungard, who explains that HSBC is continuing to refine its approach.
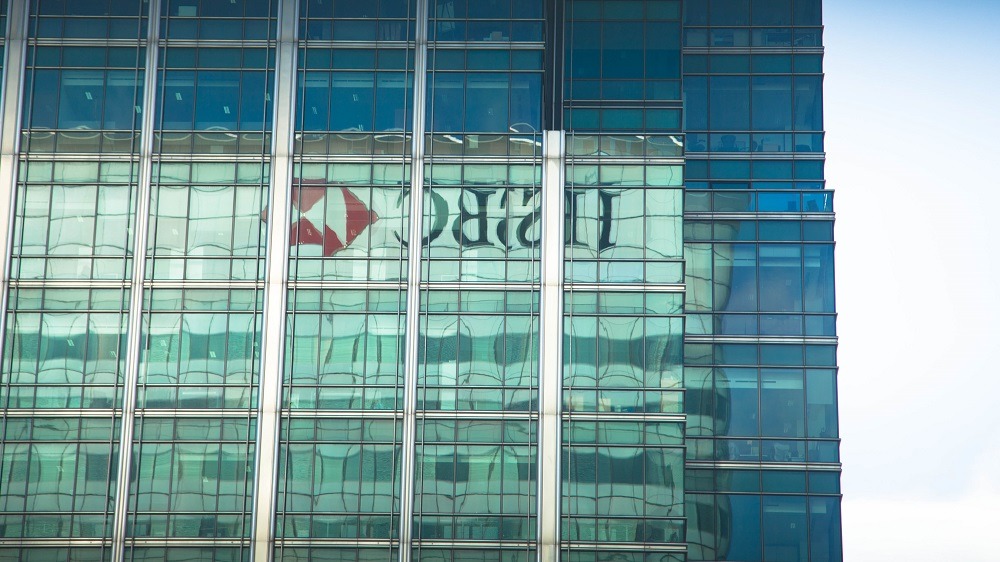
He may appreciate Celent’s recognition, but Fungard doesn’t tend to think of anti-money-laundering developments in terms of discrete steps. “You see this a lot in research analytics,” he says.
“We want to see if we can use a concept, and then we’re going to go through a testing period to see if it actually makes sense and is robust before starting to industrialise it.
“We’ve got a lot of discussions going on with regulators, and having a more modular approach gives us a chance to take lots of feedback and input from them, as well as our employees, colleagues and other external stakeholders.”
In fact, Fungard thinks the best approach to research and development cascades benefits back onto existing systems, rather than drawing a sharp dividing line between the old and the new. “It’s not that you’ve got this thing that works and does what it needs to but could be marginally better, and so you’re off building something else,” he concludes.
“As we’re developing and experimenting with new techniques, we see some things that can apply to the systems we’ve got now and improve them along the way. It’s better for now, and it’s better for the future as well.”
Tune in next week, perhaps the same will hold true for TV cops.
This article originally appeared in the summer 2019 edition of Future Banking. The full issue can be viewed here.